neXt Future Factories
New and Emerging X Technologies
This website archives the scientific production of the neXt research team at the University of South Carolina’s McNAIR Aerospace center.
About Future Factories!
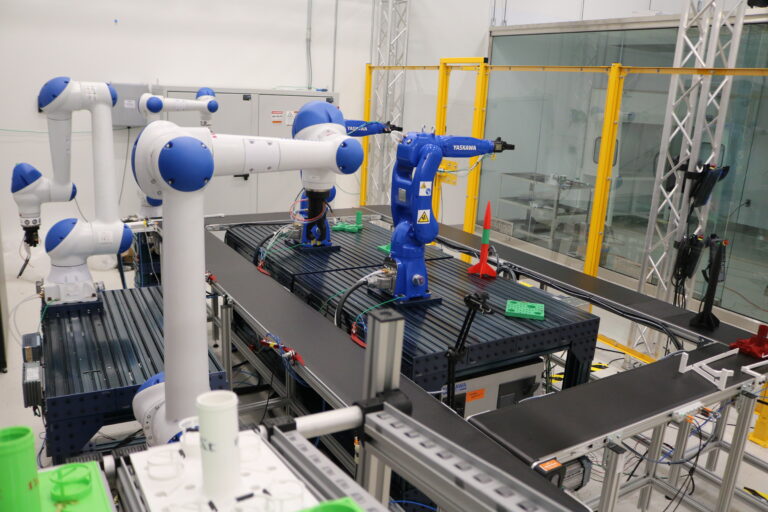
Intelligent Manufacturing Lab
Smart Manufacturing lab is a branch of Future Factories that focuses to develop an AI-driven robotic assembly cell. Advances in the cell include higher throughput, safer human intervention, self-monitoring manufacturing cells, and more autonomous operation control and scheduling.
Advanced Composites
The Advanced Composites is a branch of Future Factories that works to create a closed-form lifecycle for composites manufacturing processes. Currently the team focuses such developments on the Automated Fiber Placement (AFP) process.
Health Life Science Lab.
Health Life Science Lab is a branch of Future Factories that focuses to develop an autonomous manufacturing cell. Advances in the cell include higher throughput, safer human intervention, self-monitoring manufacturing cells, and more autonomous operation control and scheduling.
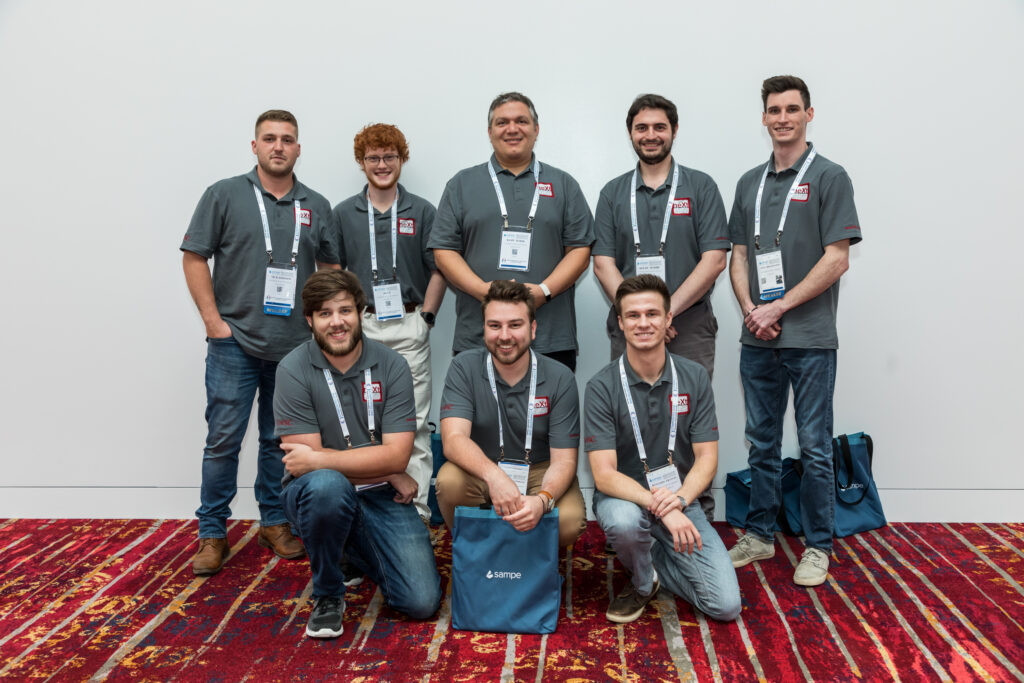
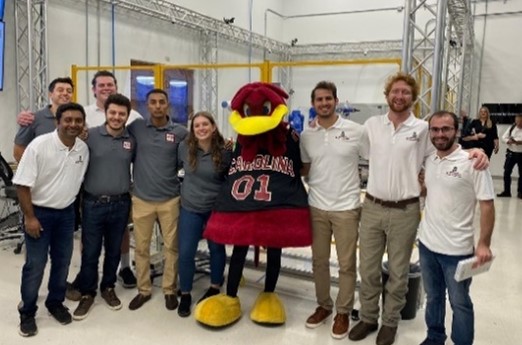
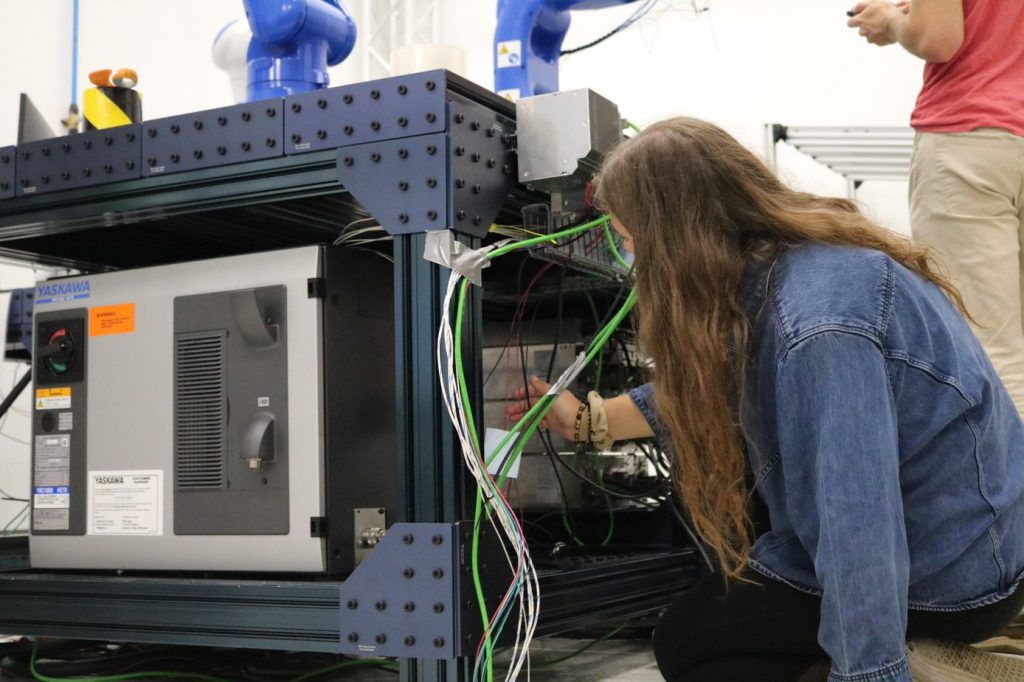
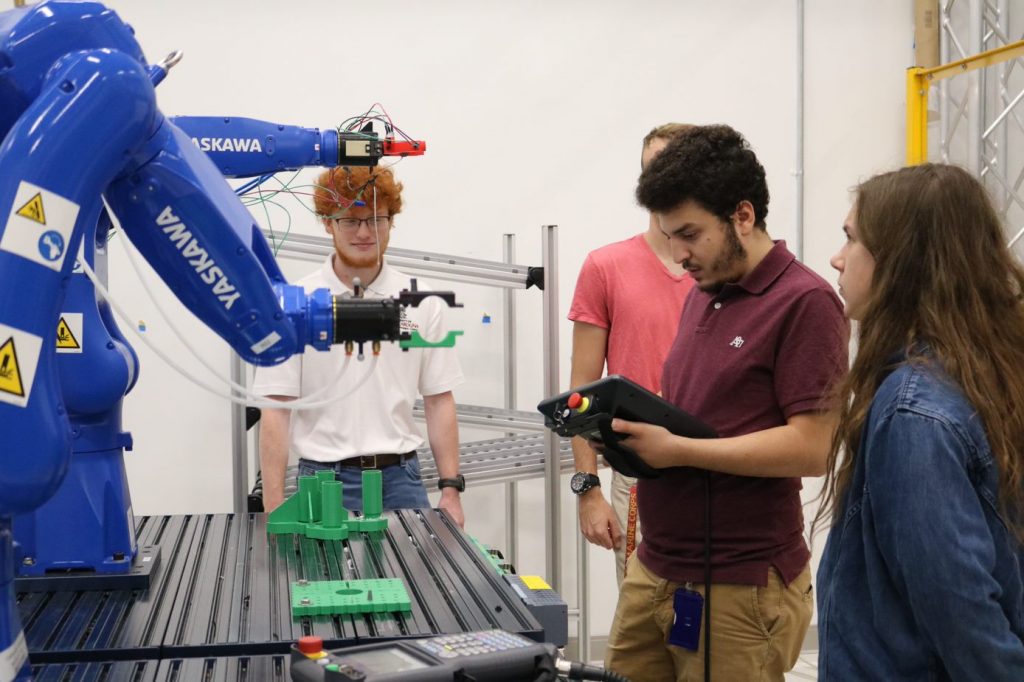
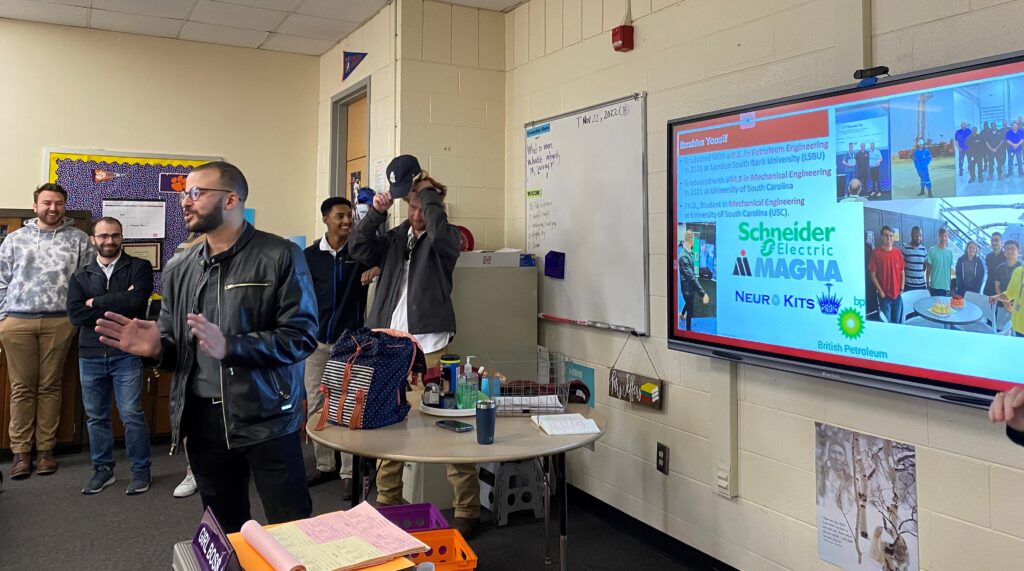
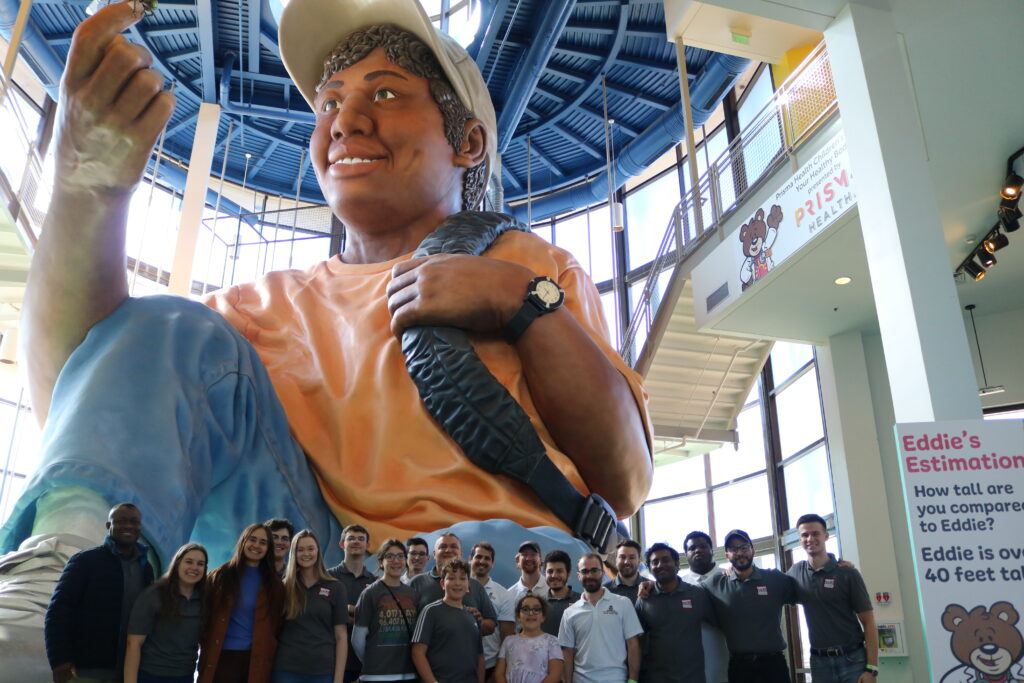
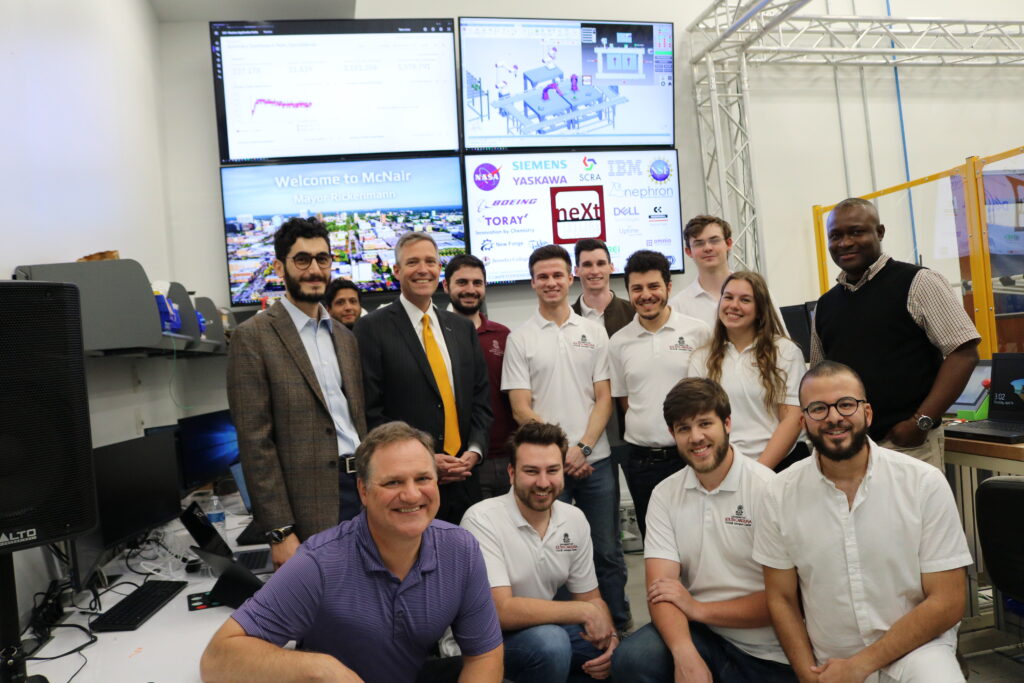
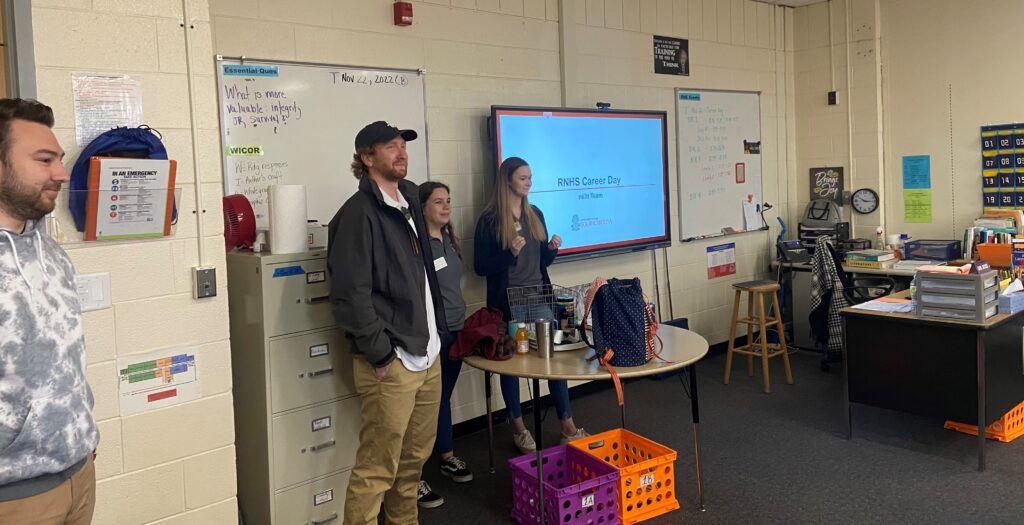
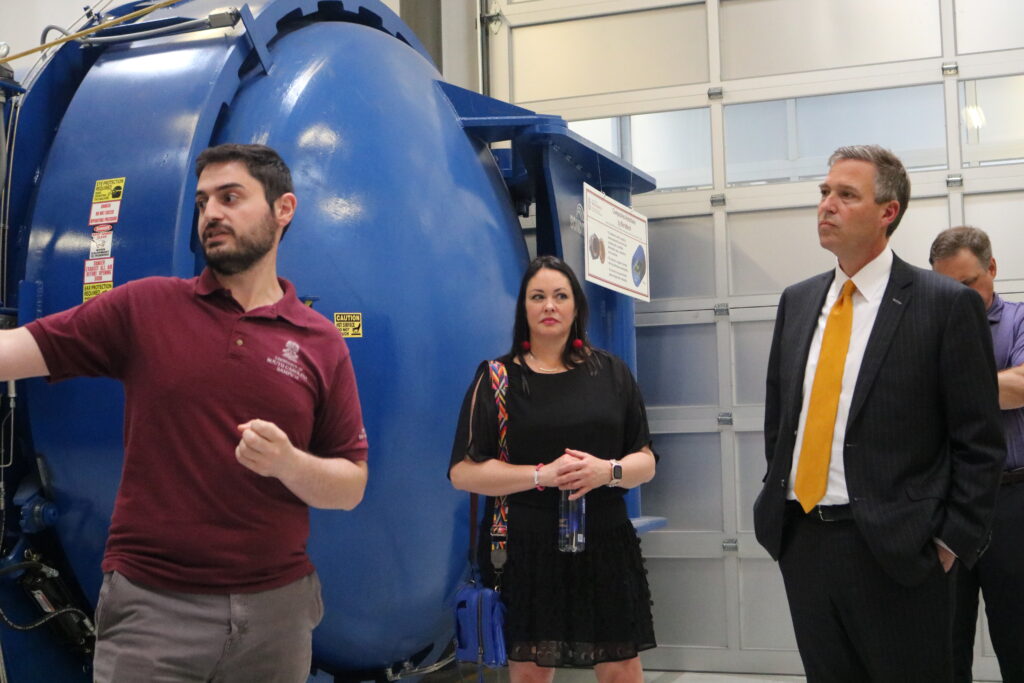
Recent Publications
-
29 Sep 2022 JournalsBayesian Optimization for Process Planning Selections in Automated Fiber Placement
-
07 Sep 2022 ConferencesSelf-sensing Smart Products in Smart Manufacturing Systems
-
06 Aug 2022 JournalsAutomated Manufacturability Analysis in Smart Manufacturing Systems: a Signature Mapping Method for Product-Centered Digital Twins
-
09 Jun 2022 JournalsA Primer on the Factories of the Future
-
24 May 2022 GrantsneXt Grant
Our Sponsors and Partnerships
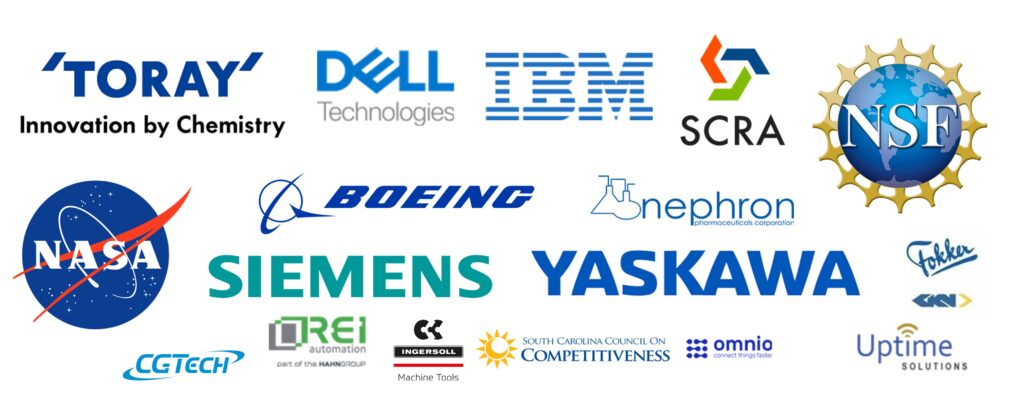
Lorem ipsum dolor sit amet, consectetur adipiscing elit. Ut elit tellus, luctus nec ullamcorper mattis, pulvinar dapibus leo.